Leveraging Data Analytics in TMS to Improve Operational Efficiency
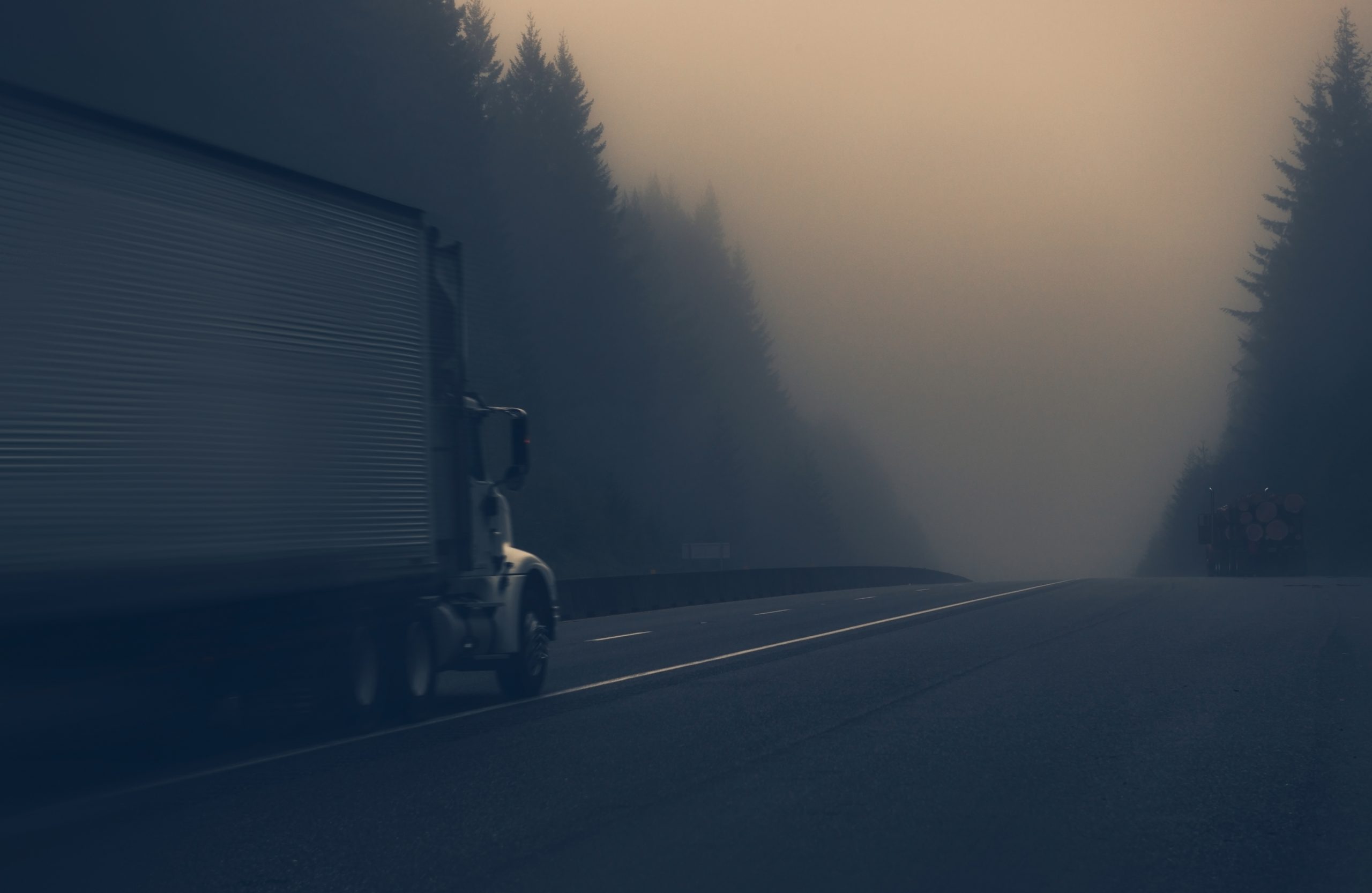
Timely deliveries, cost efficiency, and operational effectiveness are essential for businesses to stay competitive. This is where data analytics plays a vital role in optimizing transportation management systems (TMS) to drive operational efficiency.
Understanding the Role of Data Analytics in TMS
Data analytics refers to the systematic analysis of data to uncover patterns, trends, and insights that can drive informed decision-making. When applied to transportation management systems, data analytics empowers businesses to make data-driven decisions that result in cost savings, streamlined processes, and improved customer satisfaction.
Furthermore, data analytics plays a crucial role in predictive modeling within TMS. By leveraging historical data and advanced analytics techniques, businesses can forecast demand, optimize routing, and improve resource allocation. This proactive approach helps organizations anticipate challenges and implement preemptive solutions, ultimately enhancing operational efficiency and customer service.
Defining Data Analytics and TMS
Data analytics involves collecting, organizing, and analyzing vast amounts of data to extract valuable insights. TMS, on the other hand, encompasses the technology and processes businesses use to manage their transportation operations, including planning, execution, and optimization.
Moreover, data analytics in TMS extends beyond internal operations to encompass external factors such as weather patterns, traffic conditions, and market trends. By incorporating external data sources into their analytics framework, businesses can enhance their decision-making processes, mitigate risks, and capitalize on emerging opportunities in the dynamic transportation landscape.
The Intersection of Data Analytics and TMS
By integrating data analytics into TMS, businesses gain a holistic view of their transportation operations, enabling them to identify areas for improvement and implement strategies to enhance efficiency. It allows for real-time monitoring and analysis of key performance indicators, enabling proactive decision-making and continuous improvement.
Furthermore, the integration of data analytics and TMS facilitates collaboration across departments within an organization. By breaking down data silos and fostering cross-functional communication, businesses can leverage insights from various sources to drive innovation, optimize processes, and deliver superior customer experiences. This collaborative approach not only enhances operational performance but also fosters a culture of data-driven decision-making throughout the organization.
The Impact of Data Analytics on TMS Operations
Data analytics revolutionizes TMS operations by enhancing decision-making processes and streamlining workflow.
Furthermore, the integration of data analytics in transportation management systems (TMS) has brought about a paradigm shift in how businesses operate. By harnessing the power of big data, companies can now delve deeper into their operations, uncovering hidden patterns and correlations that were previously undetectable. This level of insight allows for proactive decision-making, as businesses can anticipate potential issues and take preemptive actions to mitigate risks and optimize performance.
Enhancing Decision-Making Processes
Data analytics provides actionable insights that enable businesses to make informed decisions. By analyzing historical data and predicting future trends, businesses can optimize routes, allocate resources effectively, and make strategic decisions that reduce costs and improve customer service.
Moreover, the implementation of data analytics in TMS operations empowers organizations to personalize their services based on individual customer preferences and market demands. By segmenting data and understanding customer behavior patterns, businesses can tailor their offerings to meet specific needs, ultimately enhancing customer satisfaction and loyalty.
Streamlining Operational Workflow
With data analytics, businesses can automate routine tasks, such as routing and scheduling, by leveraging algorithms and machine learning models. This not only reduces manual effort but also ensures the most cost-effective and time-efficient routes are chosen, resulting in streamlined operations and improved productivity.
Additionally, the real-time monitoring capabilities enabled by data analytics allow for dynamic adjustments to be made on the fly. By continuously analyzing incoming data streams, businesses can adapt to changing conditions, such as traffic congestion or weather disruptions, ensuring optimal efficiency and minimal disruptions to operations.
Key Data Analytics Techniques for TMS
While there are various data analytics techniques applicable to Transportation Management Systems (TMS), two essential techniques are predictive analytics and descriptive analytics. These techniques play a crucial role in enhancing operational efficiency and decision-making within the logistics and supply chain industry.
Predictive Analytics for Future Planning
Predictive analytics leverages historical data and statistical models to forecast future outcomes, such as demand fluctuations and transportation costs. By using predictive analytics, businesses can anticipate future needs and plan accordingly, optimizing their inventory levels, route planning, and resource allocation. This proactive approach enables companies to streamline their operations, reduce costs, and improve customer satisfaction by ensuring timely deliveries.
Descriptive Analytics for Real-Time Insights
Descriptive analytics focuses on providing real-time insights into ongoing operations. It involves generating reports and visualizations that display key performance indicators, such as on-time delivery rates and fuel consumption. These insights allow businesses to monitor their operations closely and make data-driven decisions to address any issues promptly. By utilizing descriptive analytics, companies can identify trends, patterns, and inefficiencies in their transportation processes, enabling them to make informed adjustments and enhance overall performance.
Moreover, the integration of advanced technologies like Internet of Things (IoT) sensors and Artificial Intelligence (AI) algorithms has further enhanced the capabilities of descriptive analytics in TMS. Real-time tracking of shipments, predictive maintenance of vehicles, and route optimization based on live traffic data are some of the innovative applications that have revolutionized the way logistics companies operate. By harnessing the power of descriptive analytics in conjunction with cutting-edge technologies, organizations can gain a competitive edge in the dynamic and fast-paced logistics landscape.
Overcoming Challenges in Implementing Data Analytics in TMS
Implementing data analytics in TMS brings numerous benefits, but it also presents challenges that need to be overcome for successful execution.
One significant challenge that organizations face when implementing data analytics in Transportation Management Systems (TMS) is the integration of disparate data sources. TMS typically gathers data from various systems, such as ERP, CRM, and supply chain management platforms. Ensuring seamless integration and data consistency across these sources is crucial for effective analytics. This challenge requires robust data governance frameworks and integration tools to harmonize data from different systems.
Addressing Data Privacy and Security Concerns
Transportation data contains sensitive information, such as customer details, shipment details, and financial data. Businesses must ensure that proper security measures, such as data encryption and robust access controls, are in place to safeguard data privacy and maintain compliance with regulatory requirements.
Moreover, with the increasing adoption of cloud-based TMS solutions, data security becomes even more critical. Organizations need to assess the security protocols of their TMS providers, implement encryption for data both in transit and at rest, and conduct regular security audits to identify and mitigate potential vulnerabilities.
Ensuring Quality and Accuracy of Data
Data analytics heavily relies on accurate, high-quality data. Businesses must have robust data management practices in place, including data cleansing, data validation, and data integration, to ensure the integrity and reliability of the data used for analysis. Without accurate data, the insights derived may be flawed, leading to ineffective decision-making.
Furthermore, ensuring data quality requires ongoing monitoring and maintenance. Organizations should establish data quality KPIs, conduct regular audits, and implement automated data quality checks to proactively identify and address any issues that could compromise the accuracy of analytical results. Data stewardship programs can also help in assigning ownership and accountability for data quality across the organization.
Measuring the Success of Data Analytics in TMS
Measuring the success of data analytics in Transportation Management Systems (TMS) goes beyond just understanding its impact on operational efficiency; it delves into the very core of how businesses optimize their transportation processes.
Implementing data analytics in TMS allows businesses to not only track the traditional key performance indicators (KPIs) like cost per mile and on-time delivery rates but also opens up avenues for more advanced metrics. These can include factors such as carbon emissions reduction, driver safety scores, and customer satisfaction levels. By incorporating a diverse range of KPIs, companies can gain a comprehensive view of how data analytics influences various aspects of their transportation operations.
Key Performance Indicators for Data Analytics
Businesses can define key performance indicators (KPIs) that align with their transportation goals. KPIs may include metrics such as cost per mile, on-time delivery rates, and inventory turnover. By monitoring these metrics before and after implementing data analytics, businesses can quantify the impact of data-driven decisions on their transportation operations.
Continuous Improvement through Data Analytics
Data analytics is not a one-time solution but a continuous journey. By regularly reviewing and analyzing data, businesses can identify trends, opportunities, and potential areas for improvement. This iterative process allows businesses to fine-tune their transportation strategies, resulting in ongoing operational efficiency gains.
Furthermore, the integration of predictive analytics into TMS can revolutionize how businesses forecast demand, optimize routes, and manage inventory levels. By leveraging historical data and machine learning algorithms, companies can proactively address challenges such as route congestion, supply chain disruptions, and fluctuating fuel prices.
In conclusion, leveraging data analytics in TMS has become a necessity for businesses seeking to improve operational efficiency in transportation management. By harnessing the power of data, businesses can make informed decisions, streamline operations, and ultimately drive competitive advantage in today’s dynamic marketplace.
Ready to Transform Your Transportation Management?
Discover how Uniq TMS can streamline your logistics operations, enhance efficiency, and boost your bottom line. Click here to learn more and explore our innovative solutions designed to meet your unique transportation needs.